Streamline the end-to-end data science lifecycle by leveraging an intuitive drag-and-drop interface for rapid model development, experimentation, and evolution. Leverage the in-built modeling algorithms to develop and deploy models on extensive datasets. Perform detailed evaluation of models through visual performance metric reports, thereby identifying, training, and optimizing for the best-fit model
AI Model Development Capabilities of NewgenONE Platform
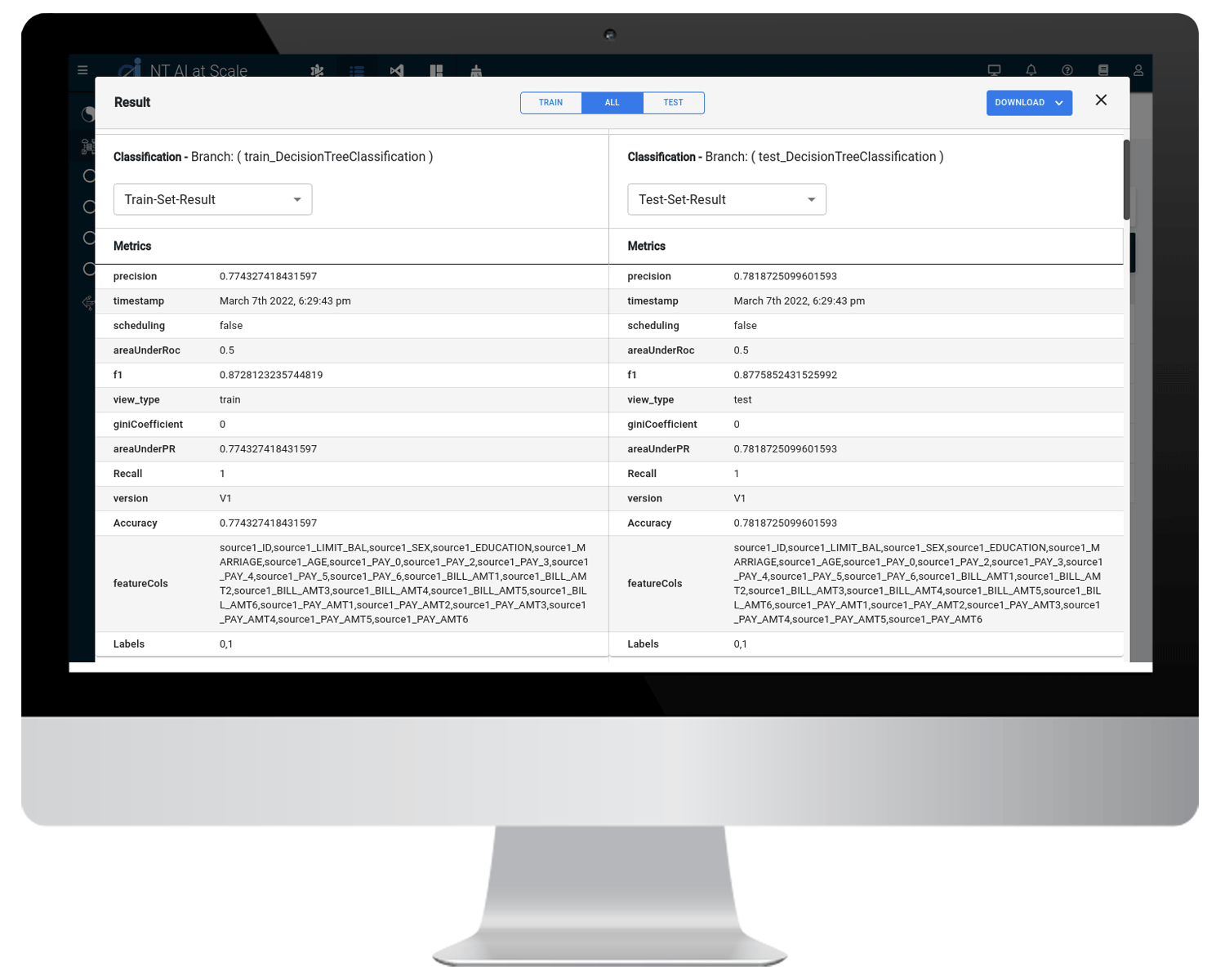
Profile Your Data for Completion, Accuracy, and Validity
Perform data profiling operations on structured and unstructured data, such as one-hot encoding, stemming, lemmatization, missing value imputation, and count vectorizer, etc.
Use built-in machine learning (ML) and deep learning-based techniques for dimensionality reduction, including singular value decomposition (SVD), principal component analysis (PCA), and restricted Boltzmann machine (RBM)
Utilize Rich Modeling Algorithms and Techniques
Use multiple options to model, including graph, ML, deep learning, and natural language processing
Perform model averaging techniques—stacking and ensembling
Develop models on massive-scale datasets by utilizing the in-memory distributed computing-based processing
Design the Model Pipeline Visually
Perform rapid model experimentation, development, and evolution through the visually intuitive drag-and-drop interface
Configure each node and drop it on the canvas with others to build your own model pipeline
Engineer Features for Supervised and Unsupervised Learning
Create and define your own features, based on separate boolean and aggregate operations with comprehensive feature engineering
Use the coding interface or the visual workflow editor to create new data columns
Access In-built Segmentation Operations
Create segments on both numeric and textual data
Create user-defined rules and conditions for segment creation
Make use of both macro and micro-level segmentation
Evaluate Models in Detail
Select the best models based on several visual performance metric reports
Evaluate the model performance using the rich set of evaluation metrics
Use multiple modeling techniques on the same feature engineered data with multi-model experimentation and evaluation
Leverage AI as a Glass Box
Access and configure all the modeling parameters
Fetch a detailed ‘feature importance report,’ explaining the output